Microsoft unveils the Phi-3 series of compact language models.
Microsoft unveils the Phi-3 series of compact language models.
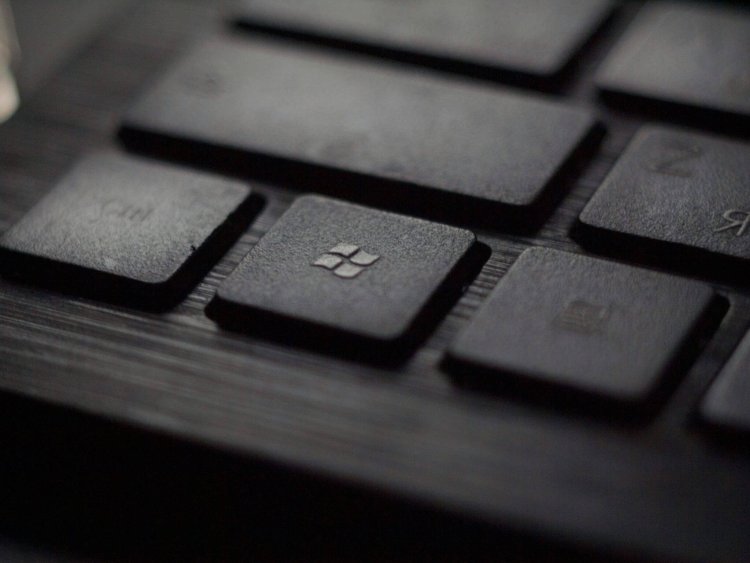
Microsoft has unveiled the Phi-3 series of open small language models (SLMs), promoting them as the most effective and economical in their class. The pioneering training methodology formulated by Microsoft's researchers has enabled these models to surpass their larger counterparts in benchmarks covering language, coding, and mathematics.
"Rather than a transition from large to small, what we're likely to see is a shift from a single type of model to a diverse portfolio, offering customers the flexibility to choose the most suitable model for their specific needs," stated Sonali Yadav, Principal Product Manager for Generative AI at Microsoft.
The premiere model of this series, the Phi-3-mini with 3.8 billion parameters, is now accessible to the public via Azure AI Model Catalog, Hugging Face, Ollama, and as an NVIDIA NIM microservice. Despite its smaller stature, the Phi-3-mini outclasses models double its size. Subsequent releases, including the Phi-3-small (7B parameters) and Phi-3-medium (14B parameters), are expected soon.
"Some clients might only require smaller models, while others might need larger ones, and many will opt to integrate both in various ways," mentioned Luis Vargas, Microsoft's Vice President of AI.
A significant advantage of SLMs is their reduced size, which facilitates on-device deployment for AI applications requiring low latency without the need for network connectivity. Potential applications include smart sensors, cameras, agricultural machinery, and more, with enhanced privacy by processing data directly on the device.
Large language models (LLMs) are adept at complex reasoning across extensive data sets, ideal for tasks such as drug discovery by analyzing interactions within scientific texts. However, SLMs present an attractive option for simpler tasks like answering queries, summarizing information, and generating content.
"Microsoft is focusing on creating tools that leverage meticulously curated data and specialized training instead of merely increasing model sizes," remarked Victor Botev, CTO and Co-Founder of Iris.ai.
"This approach not only enhances performance and reasoning capabilities but also avoids the extensive computational expense associated with trillion-parameter models. Achieving this could significantly lower the barrier to adoption for companies seeking AI solutions."
A novel data filtering and generation technique, inspired by children's bedtime stories, has been crucial to the improved quality of Microsoft's SLMs.
"Rather than solely relying on raw web data, why not seek out extremely high-quality data?" proposed Sebastien Bubeck, Microsoft's Vice President leading SLM research.
This insight led Ronen Eldan to create a 'TinyStories' dataset, comprising millions of simple narratives, by prompting a large model with vocabulary familiar to a four-year-old. Impressively, a 10 million parameter model trained on this dataset can produce fluent stories with impeccable grammar.
Building upon this initial success, the team gathered high-quality web data with significant educational value to compile the 'CodeTextbook' dataset, through cycles of prompting, generation, and filtering by both humans and large AI models.
"A rigorous selection process is integral to the production of these synthetic datasets," Bubeck noted. "We are selective with the data we use."
This high-quality training data has been transformative. "By using material akin to textbooks, we simplify the task for the language model to comprehend and interpret the content," explained Bubeck.
Despite the careful curation of data, Microsoft continues to implement rigorous safety measures with the launch of the Phi-3, mirroring the protocols established for all its generative AI models.
"In line with our standard practices for releasing generative AI models, our product and responsible AI teams employ a comprehensive strategy to identify and mitigate potential risks in the development of the Phi-3 models," stated a company blog post.
This involves additional training to reinforce appropriate behaviors, vulnerability assessments through red-teaming, and the provision of Azure AI tools to help customers develop reliable applications based on the Phi-3.